Gautam Rajendrakumar Gare
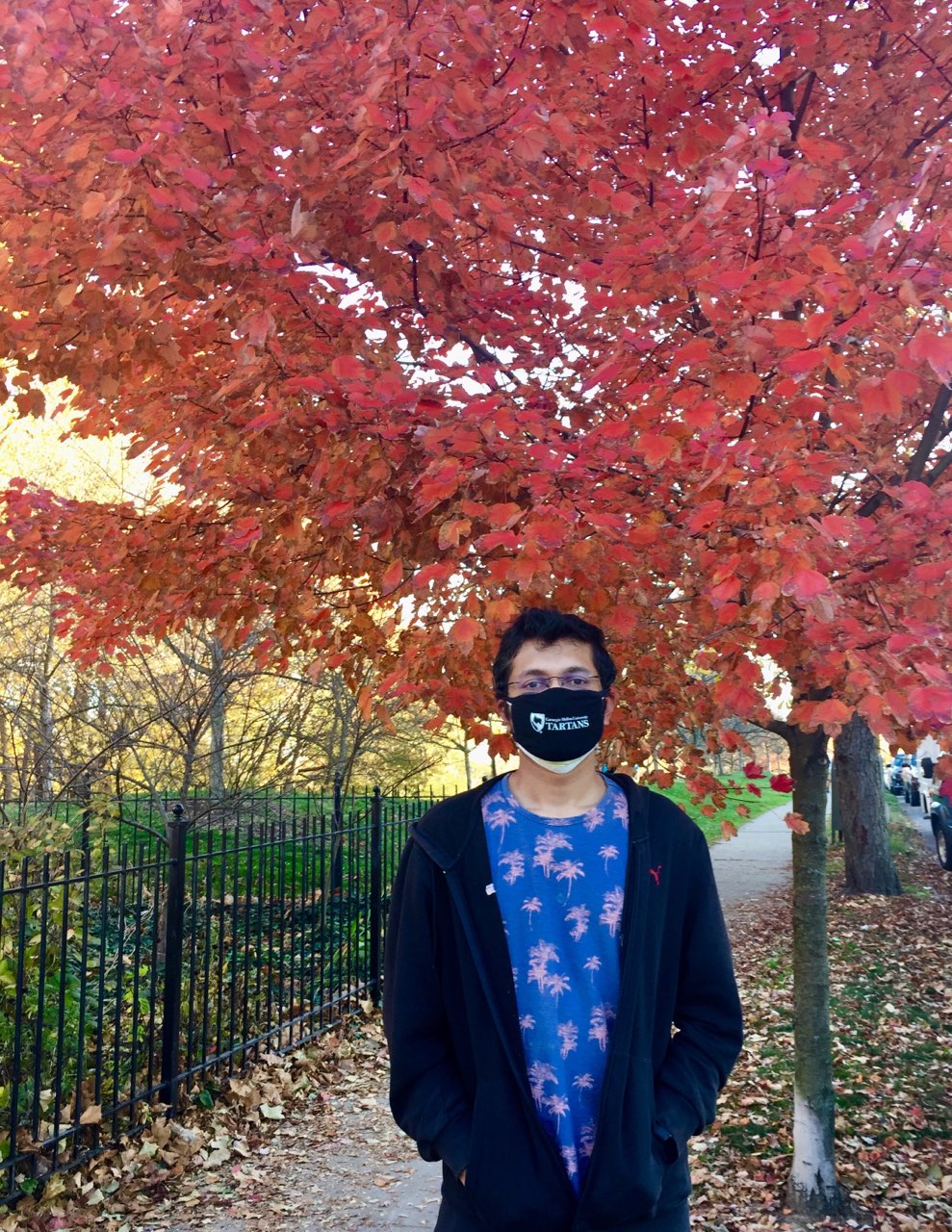
CMU Email: ggare AT andrew DOT cmu DOT edu
NSH 1502C
5000 Forbes Avenue
Pittsburgh, Pennsylvania 15213
I am a third-year Ph.D. student in the Robotics Institute at CMU, advised by Prof. John Galeotti and Prof. Deva Ramanan.
Research Interests
My research interests are in the area of Computer Vision and Deep Learning with a focus on applying causal techniques to help improve interpretability and reliability of neural networks for medical image analysis.
I obtained my master’s degree in Electrical and Computer Engineering at CMU in December 2020, wherein I carried out research under Prof. John Galeotti, Prof. Aswin C Sankaranarayanan, and Prof. Deva Ramanan. Prior to that, I got my bachelor’s degree in Electronics and Communication Engineering at B. M. S. College of Engineering, India.
I worked as a Software Engineer and Data Scientist at Sling Media subsidiary of Dish Networks, a consumer electronics company for 3 years.
News
Feb 1, 2022 |
Our paper W-Net: Dense and diagnostic semantic segmentation of subcutaneous and breast tissue in ultrasound images by incorporating ultrasound RF waveform data is published at
Medical Image Analysis journal! ![]() ![]() |
---|---|
Jul 16, 2021 |
Our paper The Role of Pleura and Adipose in Lung Ultrasound AI got accepted at
LL-COVID19 workshop MICCAI 2021! ![]() ![]() |
Jun 1, 2021 | Started my research intern at DawnLight Inc., working on Human Action recognition in medical and assisted living setups, under manager Hadi Kiapour while being advised by Prof. Fei-Fei Li and Prof. Ehsan Adeli. |
Selected publications
-
MIA 2022W-Net: Dense and diagnostic semantic segmentation of subcutaneous and breast tissue in ultrasound images by incorporating ultrasound RF waveform dataMedical Image Analysis 2022
-
LLCOVID 2021The Role of Pleura and Adipose in Lung Ultrasound AIClinical Image-Based Procedures, Distributed and Collaborative Learning, Artificial Intelligence for Combating COVID-19 and Secure and Privacy-Preserving Machine Learning 2021
-
arXivLearning Generic Lung Ultrasound Biomarkers for Decoupling Feature Extraction from Downstream Tasks2022
-
arXivExploiting Class Similarity for Machine Learning with Confidence Labels and Projective Loss Functions2021